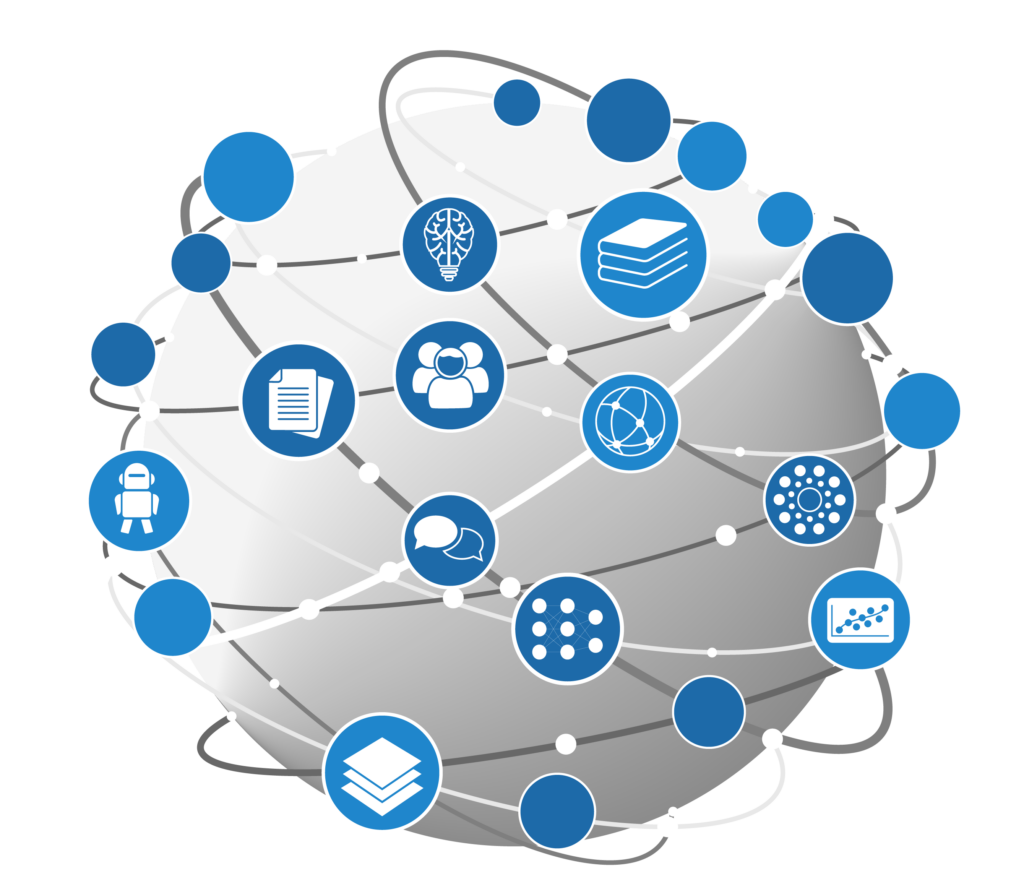
His inspiring talk summarized the evolution from Deep Learning to today's large linguistic models (LLMs), such as ChatGPT, while highlighting the challenges and potentials that await us in the coming years.
Extension of LLMs to image data
On the one hand, this approach solves the problem of limited text, since there is much more image data than text. On the other hand, images can easily be generated by observing the real world, while texts can only be written by humans. Similar to LLMs, Yann LeCun expects the emergence of a "foundation model", i.e., a model that builds emergent world knowledge about images and can be easily "refined" for specific applications.
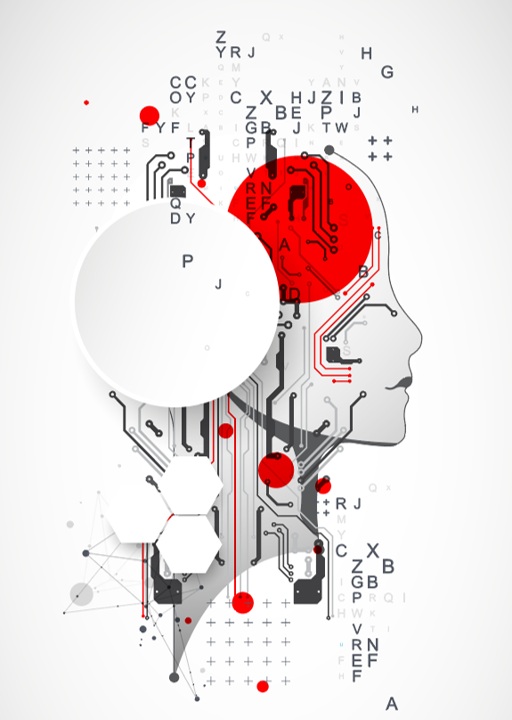
Hierarchical networks for planning thinking
Our conclusion
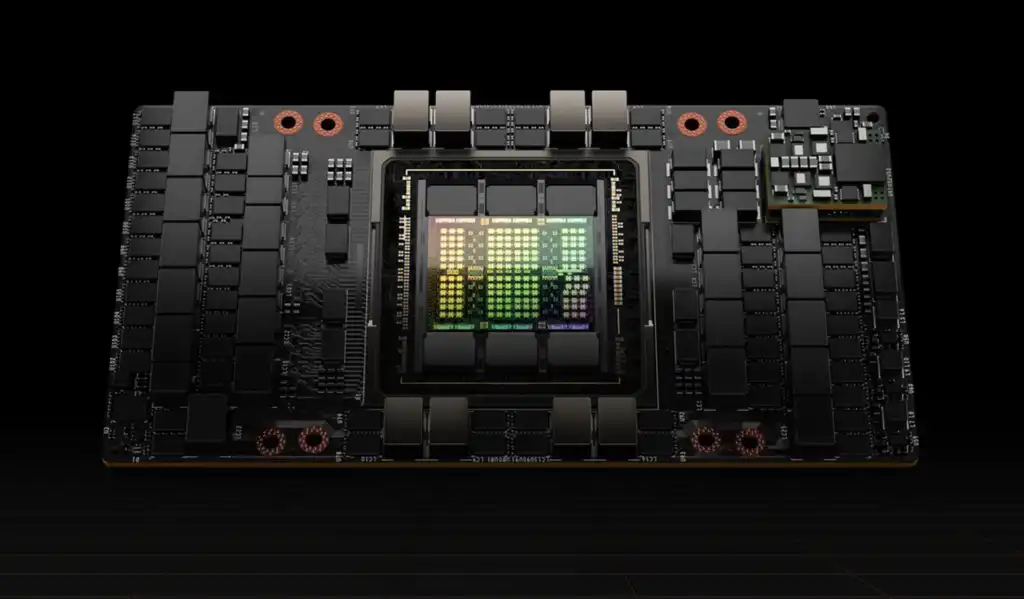
With our new nVidia H100 GPUs, at CIB we are ideally positioned to track and evaluate such issues in our research department and make the most of them in our products.
Let´s CIB!